Marcelo Bertalmío, Ph. D.
Spanish National Research Council (CSIC).
Breaking the limits of the standard model of vision
Abstract: The fields of vision science, brain modeling and machine learning with artificial neural networks (ANNs) share the same standard model (SM) abstraction, where we try to represent arbitrarily complex processes as cascades of linear filters and nonlinearities. But despite all their successes, these disciplines also share a fundamental weakness: for some basic phenomena the existing models fail unless they change with the input, but it isn’t known how to make the models self-adaptive to arbitrary inputs. The main reason behind these failures is that the SM was originated as a simplified model for single neurons that, time has shown, was ignoring biological properties that we now know are fundamental to be able to predict neural responses. Through our work developing color and contrast enhancement algorithms based on visual perception, we were able to make an interesting connection between image processing and computational neuroscience. This led us to propose the intrinsically nonlinear receptive field (INRF) formulation, a neural summation model that, unlike the SM, considers the nonlinear, dynamic and input-dependent nature of dendritic computations. We will present a number of results showing that: (1) vision models based on INRF are able to explain experimental data from visual perception and visual neuroscience that remain a challenge for the SM; (2) ANNs with INRF elements have very important advantages over their SM counterparts: they learn faster, need less training data and are more robust to input perturbations. Therefore, we believe that INRF opens up opportunities for vision research as a new building block with which to replace the linear-nonlinear elements of the SM and produce perceptual and neural models that perform better in predicting the responses to natural stimuli.
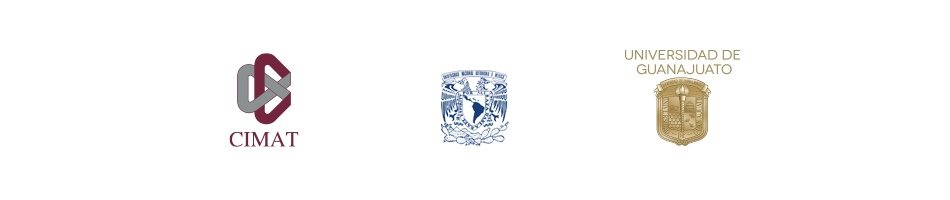